Header image: KF in DALL-E & edits in Gimp
Until recently, when I saw the abbreviation AI, I thought of Academic Integrity. Now see it more often referring to “Artificial Intelligence”. For this post, I’m thinking about “Artificial Intelligence” as artificial learning (AL), arguing that LLM output often only offers a resemblance of learning: a substitute for, a simulation of, the real thing. Now, I don’t know a lot about this field just yet. These are just some ideas I’m playing with, but I do have a lot of feelings about GenAI, and I want to get these off my chest. I hope to have some conversations about what I write so I can explore the issues further. I will likely add some updates at the bottom of the page.
Chicken : chicken nuggets = personal output : AI output
The “integrity” part of academic integrity has always stood out for me. I visualize Venn diagrams of intersecting identities, roles, ethics, dispositions, attitudes, and beliefs; the more alignment between these, the more “integrity” a person has. It suggests a coherence between what people say and do. A cohesion between what people say they believe and what they actually believe. An undividedness between the personal/professional and public/private. A unification in thought, word, and deed. Unity – wholeness – integrity.
One metaphor I am developing about LLMs is likening them to chicken nuggets. With the acknowledgment that “all metaphors are wrong but some are useful“, I offer the following:
Chicken nuggets are often made from an agglomerated paste of an unknown, and perhaps unknowable, number of chickens. I use the plural here as I’m not referring to “an amount of chicken muscle tissue” (aka chicken), but an assemblage of different parts of different chickens – Nervous tissue, connective tissue, bones, tendons, and fat – reconstituted to resemble chicken. The addition of other ingredients helps to add a reassuring texture, holding the disparate pastes together, and providing the necessary integrity needed to be able to pass chickens off as chicken. Now there are high-end chicken nuggets with a high chicken content; if I am shopping, these will be flagged by the higher price and, upon inspection, by the ingredients. Still, even these are still assemblages of chickens rather than chicken.
Typing this, Zonker’s (2006?) seminal Chicken paper comes to mind.
- The paper: https://isotropic.org/papers/chicken.pdf
- The presentation (4 mins): https://www.youtube.com/watch?v=yL_-1d9OSdk
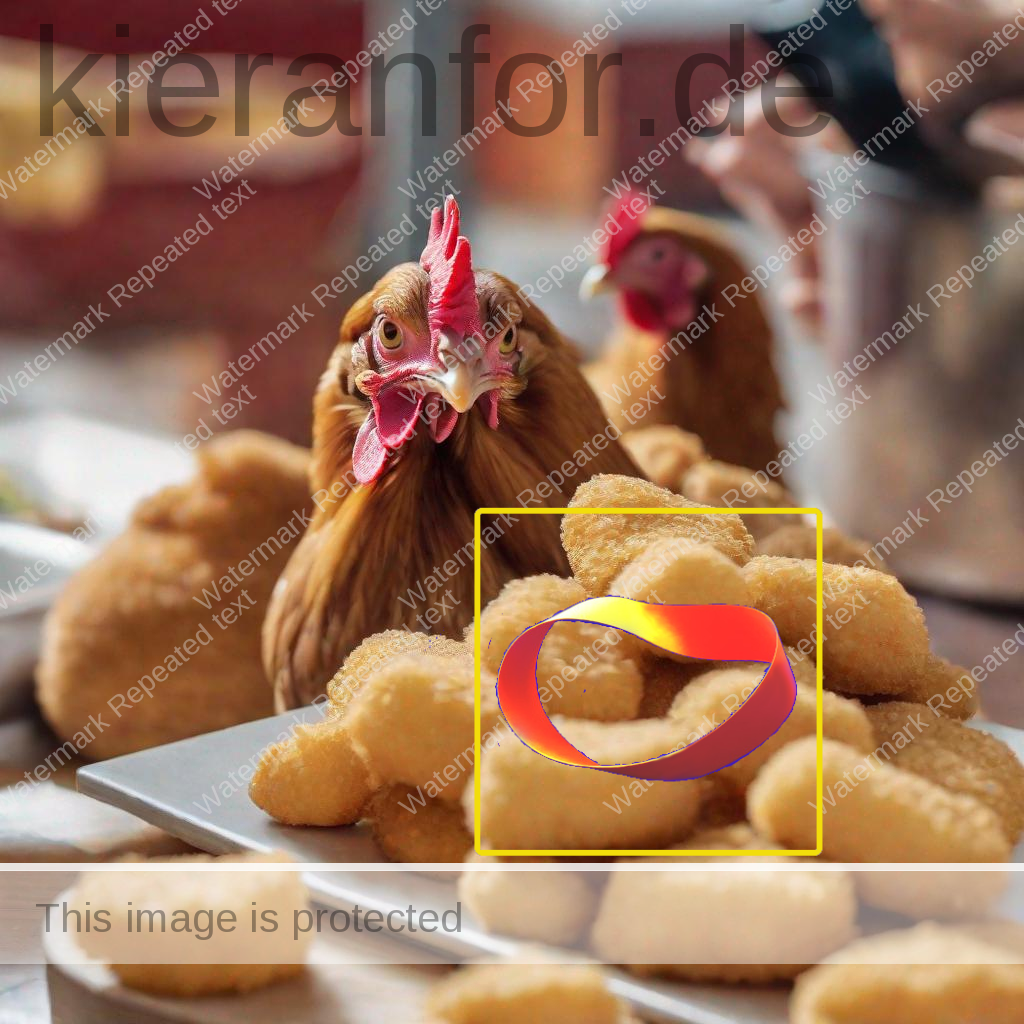
Of course, there are also cheap (cheep 🐤 ) chicken nuggets that contain a very small amount of chickens/chicken; the bulk is made up of water/fillers and preservatives. Still, these are also processed and presented to resemble chicken, though the price and ingredients will belie this.
Contrast this with a dish where the whole bird is present. This chicken is a different beast; a singular one. With all the parts still connected to each other, we are reassured of the integrity of the dish. Indeed, presented in this way, it is greater than the sum of its parts as the purchasing, preparation, and presentation of the dish can be ascribed to an actual person (or persons). Indeed, one might readily be able to trace such an animal back to its production, thus tracing a line from farm/factory to plate.
- The same logic applies to a dish of chicken breast, wings, thighs, etc. While this meat may not all be from the same animal, we can at least know how many animals were involved.
I’ll stop here lest I break the metaphor, if I haven’t already, but the imagery is simple: chicken nuggets lack the coherence of a dish where the number of chickens involved is known/knowable.
╬
The parallel here then is to consider the difference between “traditional human-generated output” and the output of LLMs.
LLMs are constructed by scraping data from online sources. As I understand it, the data is agglomerated as data sets used to train AI models; it is mixed together as a paste such that the sources of the data become unknown and unknowable. The output of this LLM is a reconstitution of this data such that it resembles data from the original sources; the appearance of coherence, of integrity, is there but it is really a simulation, a pastiche, of what was once attributable to specific sources. There are no ingredients on the box to know where the data came from and it can be very tempting to accept this output as knowledge rather than data that has been assembled to resemble knowledge. Given our susceptibility to automation bias, one may even be deceived into mistaking such output for wisdom.
Contrast this with, for example, “traditional human-generated output” such as a student paper. Here we can attribute the work in question to a specific individual. Yes, it too is an assemblage of information from different sources but the nature of its presentation makes it greater than the sum of its parts because the student’s own contributions, especially the attribution of sources, provide cohesion and integrity. The paper is the product of a long process involving an alignment of dispositions and skills. The student can account for the process, and can speak to the “ingredients”, to the production, and to the presentation.
╬╬
This issue of machine unlearning and the connection between LLMs, Machine Learning, and the Right to Be Forgotten is something I need to explore in greater detail. Trying to remove/extract parts of one particular chicken from a chicken nugget is likely extremely challenging if not impossible. With LLMs, the lack of “integrity” may mean that the agglomerated datapaste makes extracting one source impracticable. As the expert below puts it: “Deletion Must Contend With Entanglement”
- (Forbes; July 6, 2023) Google Announces Machine Unlearning Challenge Which Will Help In Getting Generative AI To Forget What Decidedly Needs To Be Forgotten, Vital Says AI Ethics And AI Law
- (APA, Jan 12, 2024) How psychology is shaping the future of technology
“We haven’t figured out the implementation of removing data from the influence and output of algorithms,” said Xu. “Machine ‘unlearning’ is a huge challenge.”
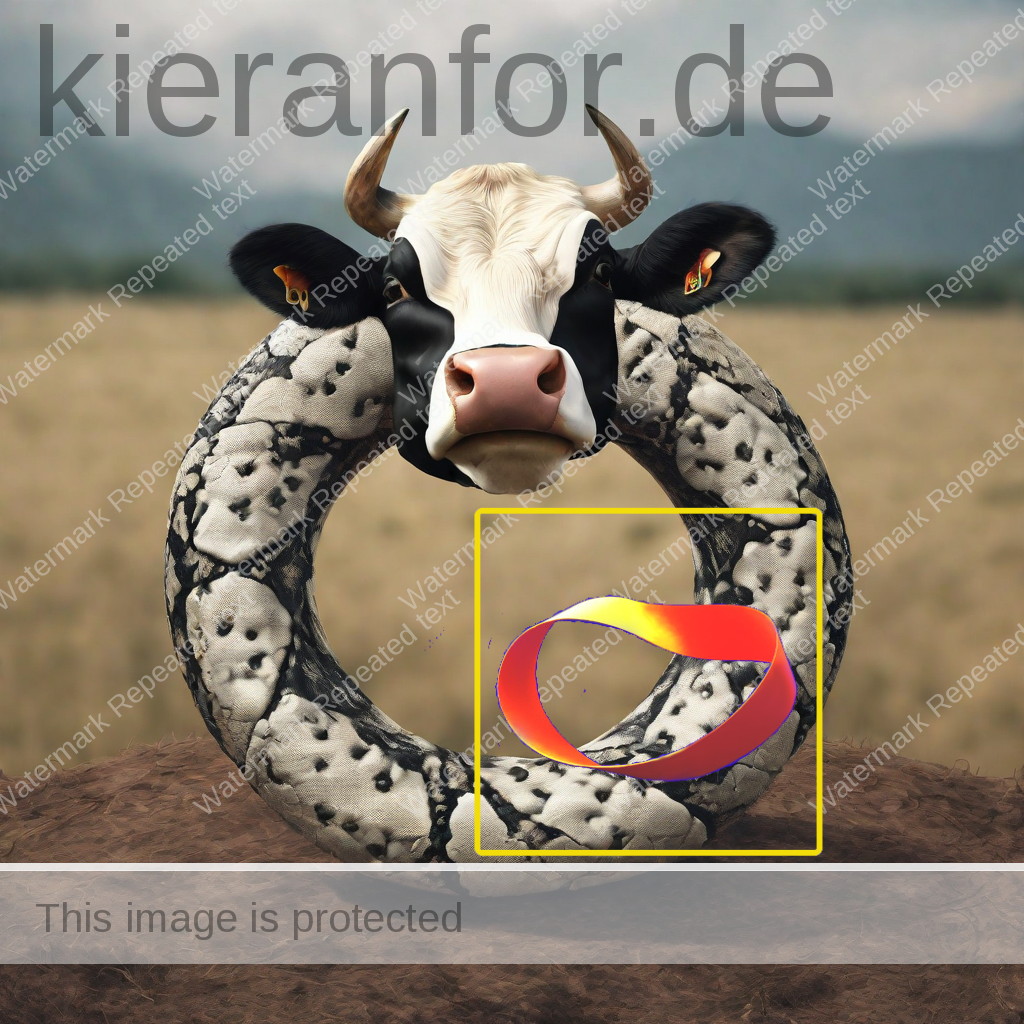
I’m also reminded of the many warnings similar to Brien Friel’s observation that “to remember everything is a form of madness“. The inability of these models to forget may cause them to get “sick” over time.
- (Aug 14, 2024) AI models ‘collapse’ and spout gibberish over time, research finds. But there could be a fix {Globe & Mail}
https://archive.is/yqgEM#selection-2548.0-2548.1
I also wonder about LLM output being fed back into LLM datasets; creating a system that essentially eats itself. This makes me think about mad cow disease (the reason I cannot donate blood in Canada. UPDATE: As of Nov 27, 2023, I am able to donate blood!) and how, as a great cartoon I can no longer find put it, “without the intervention of man, a cow would never have eaten a sheep“. Mad cow disease is thought to have been spread by cows being given feed containing the remains of other cattle who had the disease (and diseased sheep) as meat-and-bone meal [think of a bovine ouroboros]. We also know this same issue, consuming prions, is a danger noted in relation to human cannibalism. In short, when a system consumes itself, it gets sick.
Shumailov, I., Shumaylov, Z., Zhao, Y. et al. AI models collapse when trained on recursively generated data. Nature 631, 755–759 (2024). https://doi.org/10.1038/s41586-024-07566-y [TLDR]
As such, the issue of machine unlearning is an existential one as the ability to remove, for example, false/libelous/unethical/private data as well as LLM output, is necessary to ensure sustainability.
╬╬
I regularly refer to a paragraph in Chapter 1 of Dewey’s (1910) How we think where he notes that
“Reflective thinking is always more or less troublesome because it involves overcoming the inertia that inclines one to accept suggestions at their face value; it involves willingness to endure a condition of mental unrest and disturbance. Reflective thinking, in short, means judgment suspended during further inquiry; and suspense is likely to be somewhat painful. As we shall see later, the most important factor in the training of good mental habits consists in acquiring the attitude of suspended conclusion, and in mastering the various methods of searching for new materials to corroborate or to refute the first suggestions that occur. To maintain the state of doubt and to carry on systematic and protracted inquiry ― these are the essentials of thinking“
This is an issue that springs to mind when I think of LLMs: The promise of an almost immediate transition from uncertainty to certainty, with nothing in between – no thinking. This sort of immediate gratification (artificial learning) offers users the illusion of a transition from ignorance to certainty, absent the “somewhat painful” intermediate step where they sit with their own thoughts and reflect on their personal and professional values, their beliefs, their biases and prejudices …. In short, it vitiates the humility needed for the work of transforming data to knowledge, and knowledge into wisdom.
This problem exacerbated the issues that Asimov, in 1980, outlined in his piece on the Cult of Ignorance. Tom Nichols explains this further in The Death of Expertise: The Campaign Against Established Knowledge and Why it Matters (2017).
- Let us recall George Carlin’s words: “Think of how stupid the average person is, and realize half of them are stupider than that.” The internet, in a short period of time, put a vast amount of information in the hands of the average person. Suddenly, the average person could move from total ignorance of a topic and, facilitated by the disinhibition effect, proceed to expound with certainty on that topic in online forums.
- It is sort of like when everyone suddenly became a photographer with the advent of camera phones. It used to be a profession that required experience, specialized (i.e. expensive) equipment, and discernment (developing photos was expensive). Now discernment is unnecessary. We can all be photographers. And doctors. And journalists. And diplomats. And lawyers. And judges. And models. No experience is necessary; technology has democratized expertise, fostering “the false notion that my ignorance is just as good as your knowledge” (Asminov, 1980).
╬╬╬╬╬╬╬╬╬╬╬╬
“Metaphors matter. People who see technology as a tool see themselves controlling it.
People who see technology as a system see themselves caught up inside it.
We see technology as part of an ecology, surrounded by a dense network of relationships in local environments”
Nardi, B. A., & O’Day, V. (1999;2000;). Information ecologies: Using technology with heart.
- I dislike framing LLMs as “tools”. As Nardi & O’Day point out above, this suggests control over the outcome of the use of the tool. With LLMs, there is no control; the output is literally pre-determined. Prompts merely retrieve that which is already in place; if [prompt], then [answer]. Writing different prompts offers the illusion of control, a simulation of learning, but these prompts can only retrieve what is already in the system. However interesting a MidJourney image or a ChatGPT output is, it needs to be kept in mind that is not the work of the person writing the prompts. It is an assemblage, a reconstitution of the work of others, others who almost certainly thought (i.e. worked through discomfort”) in order to create that work. Anaolgies of taking steroids for fast gains spring to mind here; yes, work is still needed but the gains are disproportionate to the effort, and the effort is the whole point. Just ask Sisyphus.
- The “tools” framing also oversimplifies the nature of LLMs. If someone insists on thinking of LLMs as tools, I suggest that they think of them as self-driving cars rather than hammers. We have a pretty clear understanding of how hammers work; a hammer is a tool. I have a very basic understanding of how self-driving cars work; a self-driving car is not a tool in the same way a hammer is a tool.
Right. That’s a start. I’ll come back to this when I’ve given it some more thought.
╬╬╬╬╬╬╬╬╬╬╬╬
I spoke at this event in October. I didn’t answer most of the questions as I had nothing insightful to offer. Instead, I spoke about the issues of consent/privacy and academic integrity in the context of GenAI. I also spoke at length about chicken nuggets and made rather a poor fist of it, so I wrote the above to atone and to help me get my thoughts straight.
(Oct 24, 2023) ChatGPT in the classroom: Expectations from students and instructors
https://greencollege.ubc.ca/civicrm/event/info%3Fid%3D1690%26reset%3D1
Versions:
1.0 Oct 10, 2023: https://archive.is/zvrBd
1.1 Oct 11, 2023: https://archive.is/EMbHq
2.0 April 7, 2024: https://archive.is/pX7la
(Nov 4, 2023) AI and the privatisation of everything (Helen Beetham)
https://helenbeetham.substack.com/p/ai-and-the-privatisation-of-everything
- “Behind the scenes, while Rishi and Elon gurned happily at the idea of everyone having an AI friend, the Department for Education was shovelling more public £millions into the Oak National Academy as ‘a first step towards providing every teacher with an AI lesson planning assistant’. An idea that does not seem to have had any safety assessment or evaluation before it was funded, and is strongly resisted by teacher unions.”
🤮
(Mar 2, 2024) AI’s craving for data is matched only by a runaway thirst for water and energy https://www.theguardian.com/commentisfree/2024/mar/02/ais-craving-for-data-is-matched-only-by-a-runaway-thirst-for-water-and-energy
(Apr 16, 2025) Lab-grown chicken ‘nuggets’ hailed as ‘transformative step’ for cultured meat
https://www.theguardian.com/environment/2025/apr/16/nugget-sized-chicken-chunks-grown-transformative-step-for-cultured-lab-grown-meat